The Challenge of Hallucination in Large Language Models (LLMs)
Are AI models doomed to always hallucinate? This is a question that plagues many artificial intelligence researchers. AI Models and Hallucinations: The tendency of AI models to generate false or nonsensical information, known as hallucinations, has become a significant concern. These hallucinations can range from harmless inaccuracies to potentially dangerous misinformation. In this article, we will explore the reasons behind these hallucinations and whether there are any potential solutions. Join us as we delve into the fascinating world of AI models and their propensity for making things up.
In the unfolding panorama of artificial intelligence, the narrative surrounding Large Language Models (LLMs), such as OpenAI’s ChatGPT, has been punctuated by a significant issue: the creation of fabricated information or “hallucinations”. These instances range from quirky, albeit false declarations — such as an unfounded relocation of the Golden Gate Bridge — to grievously misleading statements that could potentially incite real-world consequences. Case in point, a recent erroneous assertion regarding an Australian mayor’s involvement in a scandal, threatened to stir legal repercussions. Furthermore, experts have discovered that the propensity of these systems to “hallucinate” can inadvertently foster the distribution of malevolent code packages, besides disseminating misinformed health and medical advice.
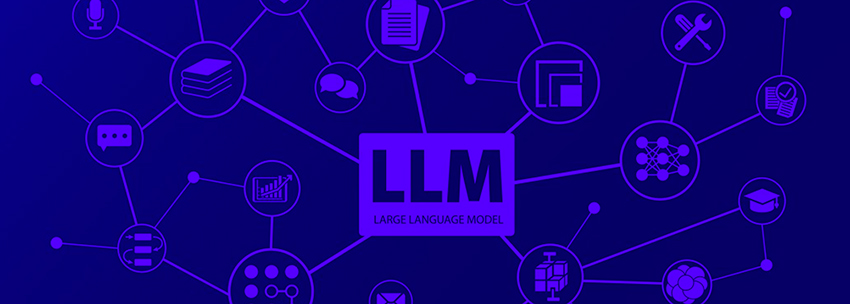
Hallucination, in the context of AI, arises from the inherent methodology applied in the training and development of these generative models. Essentially devoid of genuine intelligence, these systems rely heavily on statistical analyses to predict subsequent strings of data, be it text, images or even music. They assimilate a vast array of examples, predominantly extracted from public internet resources, to comprehend patterns and contextual relationships within the data.
At the core, Generative AI models operate devoid of any inherent intelligence. Rather, they function as advanced statistical engines capable of predicting a vast array of data formats including but not limited to text, imagery, speech, and music. These models hone their predictive prowess through the ingestion of an immense volume of examples, primarily harvested from the expanses of the public web. In doing so, they become adept at recognizing likely data patterns and associations within a given contextual backdrop.
Consider the instance of crafting a response to an email which signs off with “Looking forward…”, an LLM, utilizing its training, could potentially complete the phrase with “… to hearing back”, a pattern it has recognized from a myriad of similar email exchanges it has processed. This, however, does not indicate any form of anticipation or forward-thinking from the AI.
Sebastian Berns, a distinguished researcher from Queen Mary University of London, elucidated that the present structure of training LLMs incorporates a masking technique, where preceding words are hidden to facilitate predictions for replacements. This approach mirrors the predictive text feature in iOS, where ongoing suggestions are generated based on past data inputs.
Despite its effectiveness in generating coherent text, this method cannot assure the veracity of the generated content. It can sometimes amalgamate information from disparate, even fictional sources, leading to content that is grammatically accurate but inherently false or nonsensical. Berns highlighted that the core of the issue is the LLM’s incapacity to gauge the reliability of its predictions, rendering it unable to discern the credibility of its responses or predictions.
As the tech community grapples with this issue, the consensus leans towards the improbability of completely eradicating hallucinations. However, Vu Ha from the Allen Institute for Artificial Intelligence posits that refining the training and deployment processes can potentially mitigate these occurrences. He emphasizes that pairing LLMs with well-curated knowledge bases can significantly enhance their accuracy, as demonstrated by the performance disparity between Microsoft’s Bing Chat and Google’s Bard when tasked with identifying the authors of a significant paper.
According to Ha, the true dilemma lies in weighing the pros and cons — assessing whether the utility of these AI systems outweighs the repercussions of occasional inaccuracies. An optimal approach would focus on maximizing the positive utility these systems can bring to the table.
Addressing this issue from another angle, OpenAI initiated a strategy called Reinforcement Learning from Human Feedback (RLHF) in 2017, aiming to curtail hallucinations. This technique entails an iterative process where LLMs are fine-tuned based on human feedback, nurturing them to generate more reliable outputs. However, Berns cautioned that this method has its limitations, particularly in fully aligning the LLM’s responses with human expectations, often yielding inconsistent results.
Berns proposes a philosophical reassessment of the situation, advocating for a more balanced perspective. He suggests that the ability of LLMs to hallucinate can actually foster creativity, serving as a catalyst for novel thought processes and ideas, especially in artistic domains. In contrast, Ha contemplates the lofty expectations set for modern LLMs, noting a kind of cognitive dissonance experienced by users when the aesthetically pleasing outputs of these models unravel upon closer scrutiny.
In essence, the path forward may not necessarily involve technical advancements but rather an adjustment in perspective, fostering a community that approaches these AI predictions with a discerning and critical mindset.
Conclusion
The issue of hallucination in large language models (LLMs) like OpenAI’s ChatGPT is a significant challenge that needs to be addressed. While it may be impossible to completely eliminate hallucinations, there are ways to mitigate them and improve the overall accuracy of LLMs.
By pairing LLMs with well-curated knowledge bases and utilizing reinforcement learning from human feedback (RLHF), we can enhance their accuracy and reliability. However, it’s important to recognize the limitations of these methods, as they may not always align perfectly with human expectations.
Moreover, rather than solely focusing on technical advancements, it is crucial for us to adopt a more balanced perspective towards LLMs. This includes fostering a critical mindset and approaching AI predictions with skepticism, especially when it comes to important or sensitive information.
Ultimately, as LLMs continue to evolve, it is essential for the AI community to work collaboratively towards finding solutions and developing guidelines that ensure the responsible and ethical use of these powerful models. By doing so, we can navigate the complex world of AI-generated content while maximizing the benefits and minimizing the risks associated with hallucinations.
How do you think LLMs’ tendency to hallucinate can be managed effectively to balance creativity and factual accuracy in AI-generated content? Do you believe that public awareness and education about the limitations of AI models like LLMs are crucial in mitigating the impact of hallucination? What role do you think ethical considerations should play in addressing hallucination in AI-generated content, particularly when it comes to misinformation and safety concerns?
Share your thoughts below!