AI2 Releases Open-Source Text-Generating AI Models and Their Training Data
AI2, the Allen Institute for AI, has made a groundbreaking move by open sourcing its text-generating AI models. In addition to sharing the models, AI2 is also providing the data used to train them, making it one of the largest public data sets of its kind. This release, called OLMo (Open Language MOdels), aims to foster research and experimentation in the field of text-generating AI. Unlike many other models, OLMo was trained on transparent and open data sets, allowing developers to use them freely for training, experimentation, and even commercialization. In this blog post, we will explore the significance of AI2’s open source approach, the capabilities of the OLMo models, and the potential impact on the AI community.
The Allen Institute for AI (AI2), established by the late co-founder of Microsoft, Paul Allen, is launching a series of generative AI language models. These models are presented as being more accessible and transparent compared to existing alternatives. Significantly, they come with a licensing framework designed to offer developers extensive freedom. This allows for a broad range of applications, including training, experimentation, and commercial use, without the usual restrictions.
Named OLMo, which stands for “Open Language Models,” these models, along with their training dataset named Dolma—one of the most extensive publicly available datasets of its kind—were developed with the goal of advancing the scientific understanding of AI’s capability to generate text. This initiative, as outlined by Dirk Groeneveld, a senior software engineer at AI2, aims to delve into the intricate science that enables AI to produce coherent and contextually relevant text.
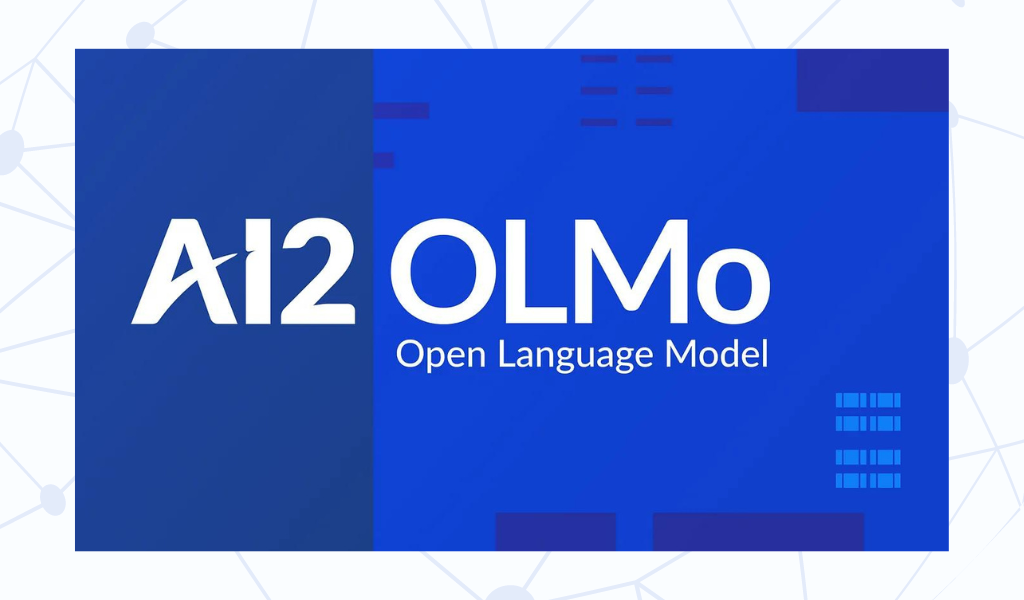
“The term ‘open’ carries multiple meanings in the context of text-generating models,” explained Dirk Groeneveld. He anticipates that the release of the OLMo framework will be eagerly embraced by both researchers and practitioners as a valuable resource. It offers access to one of the most substantial public datasets ever made available, accompanied by all the essential components needed for model development. This opens up new possibilities for in-depth analysis and innovation in the field of generative AI.
The landscape of open-source text-generating models is becoming increasingly crowded, with entities ranging from Meta to Mistral unveiling sophisticated models available for developers to adapt and refine. However, Groeneveld argues that the ‘open’ label might not fully apply to many of these models. His contention is based on the fact that their development often occurs in private, utilizing datasets that are proprietary and not transparent, which can limit the true openness and accessibility of these models for wider research and development purposes.
In stark contrast, the OLMo models stand out for their transparency and collaborative development, having been created with contributions from partners such as Harvard, AMD, and Databricks. These models are accompanied by the actual code used to generate their training data, in addition to comprehensive training and evaluation metrics and logs. This level of openness ensures that users not only have access to the models themselves but also to the detailed processes and methodologies that underpin their creation, facilitating greater reproducibility and trust in the models.
Regarding effectiveness, Groeneveld highlights that the premier OLMo model, OLMo 7B, presents a robust and competitive option compared to Meta’s Llama 2, albeit with some application-specific nuances. In certain evaluations, especially those focused on reading comprehension, OLMo 7B demonstrates a slight advantage over Llama 2. However, in other areas, such as question-answering tasks, it lags marginally behind. This nuanced performance underscores the importance of choosing the right model based on the specific requirements and goals of the application in question.
The OLMo models exhibit certain constraints, such as suboptimal performance in non-English languages, largely because the Dolma dataset predominantly comprises English content, and their capabilities in generating code are not as strong. However, Groeneveld emphasizes that this is just the beginning. These early limitations are part of the iterative process of AI development, suggesting that there is significant potential for improvement and adaptation as the models evolve and more diverse datasets are incorporated.
“Currently, OLMo hasn’t been tailored for multilingual use,” Groeneveld clarified. “Furthermore, while code generation wasn’t the central focus of the OLMo framework at this stage, we’ve included approximately 15% code in OLMo’s data compilation to lay the groundwork for future projects that may involve fine-tuning for code-related tasks.” This approach indicates a strategic foresight in the development of OLMo, ensuring it has the foundational elements to expand its capabilities in code generation and multilingual support in the future.
Groeneveld was questioned on the potential for the commercially usable and highly efficient OLMo models, which are capable of operating on consumer-grade GPUs such as the Nvidia 3090, to be exploited for unintended or malicious purposes. Concerns arise in light of findings from a study conducted by Democracy Reporting International’s Disinfo Radar project. This initiative, dedicated to identifying and mitigating trends and technologies in disinformation, discovered that widely used open text-generating models, including Hugging Face’s Zephyr and Databricks’ Dolly, could consistently produce harmful content when given malicious prompts, showcasing a tendency to create “imaginative” toxic responses.
Groeneveld maintains that the advantages of developing an open platform ultimately surpass the potential drawbacks.
“Creating this open platform will actually enable more extensive research into the potential dangers of these models and strategies for mitigating them,” he explained. While acknowledging the risk that open models might be misused or applied in ways not originally intended, Groeneveld emphasizes that an open approach fosters technological progress towards more ethical AI models. He argues that transparency is essential for verification and reproducibility, as these can only be accomplished with full access to the underlying technology. Furthermore, he highlights that this methodology helps to decentralize the concentration of power in the field, thereby facilitating more equitable access to advanced AI technologies.
Over the next few months, AI2 is set to unveil more advanced and larger OLMo models, encompassing multimodal models that can process and understand various forms of data beyond text. Additionally, they will provide new datasets to support the training and fine-tuning of these models. In keeping with their commitment to accessibility and open research, AI2 will continue to offer these resources at no cost on platforms such as GitHub and Hugging Face, a popular repository for AI projects. This move aligns with AI2’s dedication to fostering innovation and collaboration within the AI community by ensuring that cutting-edge tools and data are readily available to researchers and developers worldwide.
Conclusion
AI2’s decision to open source its text-generating AI models and provide the accompanying data sets marks a significant milestone in the field of AI research. By making these resources freely available, AI2 is not only promoting transparency and collaboration but also empowering developers to explore new possibilities and push the boundaries of text generation. While the OLMo models have their limitations, they serve as a strong alternative to existing models and pave the way for future advancements.
Furthermore, AI2’s commitment to addressing the potential risks and promoting ethical use of these models demonstrates their dedication to creating a more equitable and responsible AI ecosystem. As AI2 continues to expand its offerings and release more capable models, the AI community can look forward to further advancements and opportunities for innovation. With the release of OLMo and Dolma on GitHub and Hugging Face, AI2 is fostering a culture of openness and accessibility that will undoubtedly shape the future of text-generating AI.
Are you excited about the potential for developers to freely use and experiment with the OLMo models and the accompanying data sets? What are your thoughts on the importance of transparency and open data sets in the development of AI models? Do you believe that the OLMo models have the potential to outperform existing models in certain applications? Why or why not? Share your insights below.