Embodied AI: Pen-Spinning and Living Room Cleaning in New Research
Embodied AI is a fascinating field that aims to teach artificial intelligence systems to interact with the real world. In recent research, tech giants Meta and Nvidia have made significant advancements in this area, focusing on practical tasks like pen-spinning and living room cleaning. By using simulated environments and innovative techniques, these companies are paving the way for AI to become more capable and useful in everyday scenarios. In this blog post, we’ll explore the exciting developments in embodied AI and how they can benefit home automation enthusiasts.
Certainly, AI can craft beautiful sonnets and even give Homer Simpson a run for his money with a Nirvana cover. However, to truly embrace our emerging technological leaders, they must prove their prowess in more practical domains. That’s precisely why Meta and Nvidia are pushing the boundaries, training their AI systems in everything from pen tricks to collaborative household chores.
Both of these tech giants happened to release new research this morning focusing on instructing AI models to engage with the physical world. They’re accomplishing this feat through ingenious utilization of a simulated environment.
As it turns out, the real world isn’t just intricate and chaotic, but it’s also slow-moving. When agents are trained to control robots and carry out tasks like opening a drawer and placing an item inside, it might necessitate repeating the task hundreds or even thousands of times. This would consume several days. However, if these agents practice in a reasonably realistic simulation of the real world, they can attain near-proficiency in just a minute or two.
Simulators have been a staple in AI research, but Nvidia has taken a step further by introducing an extra layer of automation. They’ve harnessed a large language model to assist in generating reinforcement learning code, offering guidance to a novice AI in enhancing its task performance. This system is known as EUREKA, short for Evolution-driven Universal REward Kit for Agent.
For example, imagine you want to instruct an AI to pick up and sort objects by their color. There are numerous ways to define and code this task, but not all methods are equally efficient. Should the robot aim for fewer movements, or should it prioritize a quicker completion time? While humans are adept at coding these tasks, determining the best approach often requires some trial and error.
Surprisingly, the Nvidia team discovered that an AI model trained in coding (LLM) excels at this, frequently outperforming humans in developing effective reward functions. What’s more, it continues to iterate on its own code, progressively improving and enabling generalization across various applications.
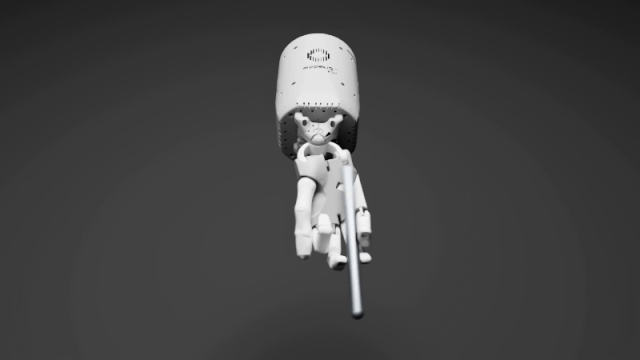
The remarkable pen trick shown above is, admittedly, a simulation, but its creation required significantly less human time and expertise than if EUREKA hadn’t been employed. Through this approach, AI agents have excelled in various virtual tasks related to dexterity and locomotion. It even exhibits proficiency in tasks like using scissors, which is, undoubtedly, quite beneficial.
Of course, transitioning these actions into real-world applications presents a distinct and unique challenge—truly embodying AI. Nonetheless, it’s a definite indicator that Nvidia’s commitment to generative AI goes beyond mere words.
Enhanced Environments for Future Robot Companions
Meta is fully invested in the development of embodied AI, and it has unveiled several notable breakthroughs today. Their journey begins with a fresh iteration of the “Habitat” dataset. The initial version made its debut back in 2019, and it consisted of a collection of 3D environments that were nearly photorealistic and meticulously annotated, offering AI agents an immersive space to navigate. While the concept of simulated environments is not new, Meta’s objective was to enhance their accessibility and ease of use.
Subsequently, they introduced version 2.0, featuring a broader array of environments that were highly interactive and remarkably true to the physical world. Moreover, they began curating a repository of objects to populate these environments, a practice that has proven valuable for numerous AI companies.
Now, with Habitat 3.0, a new dimension is introduced: human avatars sharing the same space via VR. This means that real people or AI agents trained to mimic human behavior can step into the simulator alongside the robot and engage with both it and the environment simultaneously.
The concept may sound straightforward, but it holds profound significance. Imagine you’re teaching a robot to tidy up the living room—moving dishes from the coffee table to the kitchen and gathering scattered clothing into a hamper. If the robot operates alone, it may develop a specific strategy that’s easily disrupted by the presence of a person nearby, who might even assist in the task.
However, when a human or human-like agent shares the space, the robot can repeat the task thousands of times within a few seconds, adapting and learning to collaborate or navigate around them. This innovative feature greatly enhances the robot’s ability to interact with real-world scenarios.
They’ve coined the cleanup task “social rearrangement,” while another vital one is known as “social navigation.” In the case of social navigation, the robot is required to discreetly trail someone, ensuring it remains within audible distance or acts as a safety observer. Think of it as a small robot companion that accompanies a person in a hospital, providing support when they need to visit the bathroom, for instance.
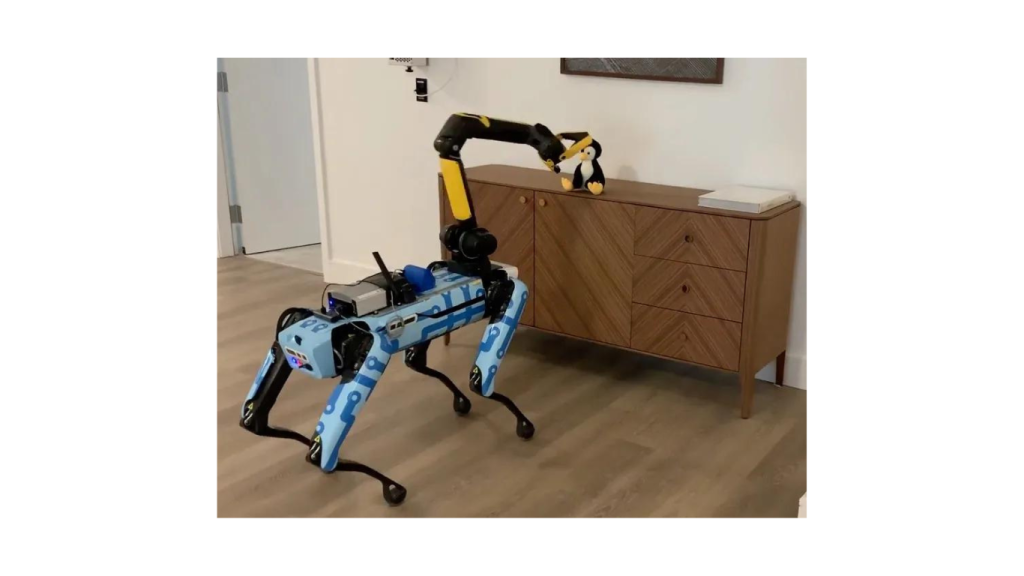
A new database of 3D interiors, referred to as HSSD-200, not only enhances the fidelity of these environments but also proved more effective during training. Surprisingly, training in approximately a hundred of these high-fidelity scenes yielded better results compared to training in 10,000 lower-fidelity ones.
Meta has also introduced a new robotics simulation stack named HomeRobot, designed for use with Boston Dynamics’ Spot and Hello Robot’s Stretch. Their intention is to standardize basic navigation and manipulation software, enabling researchers to concentrate on more advanced areas where innovation is in demand.
Both Habitat and HomeRobot are available under an MIT license on their respective GitHub pages, while HSSD-200 is accessible under a Creative Commons non-commercial license. Researchers, feel free to explore and utilize these resources.
What safety protocols are in place to ensure the safety of the AI while it is performing tasks?
Ensuring the safety of AI while it performs tasks is a critical concern. Both Meta and Nvidia are taking precautions to address this issue.
Nvidia’s EUREKA system employs reinforcement learning to train AI agents in simulated environments. This allows the AI to practice and learn tasks without the risk of physical harm to itself or others. By using virtual simulations, the AI can repeat tasks hundreds or thousands of times to improve its performance. This iterative process helps the AI generalize its learning to different applications.
Meta, on the other hand, has developed the Habitat dataset, which provides carefully annotated 3D environments for AI agents to navigate. With the introduction of version 3.0, human avatars can now interact with the AI agents in these simulations. This allows the AI to learn how to work with or around humans, enhancing its safety and adaptability in real-world scenarios.
Additionally, both Meta and Nvidia prioritize safety in their research and development processes. They conduct extensive testing and validation to minimize the risk of accidents or unintended consequences. They also comply with ethical guidelines and regulations to ensure responsible AI development.
While these safety protocols are in place, it’s important to note that embodying AI in the physical world presents unique challenges. The integration of AI into real-world environments requires additional safety measures, such as implementing safety sensors, fail-safe mechanisms, and rigorous testing before deployment.
Overall, Meta and Nvidia recognize the importance of safety in AI systems and are committed to developing technologies that prioritize the well-being of both humans and AI agents. They continuously work towards enhancing safety protocols and ensuring responsible AI development.
Conclusion
In conclusion, the recent research conducted by Meta and Nvidia on embodied AI, focusing on tasks like pen-spinning and living room cleaning, showcases the significant progress being made in teaching AI systems to interact with the real world. By utilizing simulated environments and innovative techniques, these advancements have the potential to revolutionize household assistance and personalized robotics, offering enhanced support, efficiency, and safety.
With AI companions capable of learning and adapting in real-world scenarios, we can expect more personalized and intelligent assistance, freeing up time and energy for individuals, while also paving the way for AI-enabled companions in healthcare and other domains. The future looks promising as embodied AI continues to transform our interactions with technology and creates a more connected and efficient world.
What are your thoughts on the advancements in embodied AI showcased by Meta and Nvidia? Can you imagine any potential ethical considerations or limitations that need to be addressed as AI becomes more integrated into our daily lives? Share your insights below.